You’ve narrowed your options. You’re ready to buy. But you still have questions.
May 19, 2025
By David Golub And Francois Silvain
You’ve narrowed your options. You’re ready to buy. But you still have questions.
This is a critical moment where shoppers begin to imagine ownership, seeking answers from brand content, expert reviews, forum discussions or in-store specialists – options that can be time-consuming and sometimes still leave questions unanswered.
Having previously assessed AI shopping agents for product discovery, we took the next step and wondered, can they also help close a sale? Can shopping bots fill the information gap by delivering on-demand product expertise exactly when shoppers need it?
Working with three high-information purchases inspired by our Icelandic trekking scenario – a backpack, a GPS device and sunscreen – we wanted to see if AI can deliver an intent-based shopping experience at a level that might disrupt traditional buying journeys, both online and ultimately in-store.
As discussed below, our short answer is yes: When content comes wrapped with customer empathy and delivers a payload of contextual awareness, agents can do a remarkable job illuminating feature distinctions, making recommendations and resolving objections.
Moreover, our review also shows that commercial LLMs are not at the same level: Perplexity in particular and ChatGPT impressed, while Amazon’s Rufus and Google Gemini fell short in delivering expert-human sales support.
We end by exploring the high stakes for brands as agentic shopping shifts the paradigm from keyword-based search to intent-driven conversational experiences, highlighting actions brands must take today to remain visible to tomorrow’s buyers.
Our Testing Methodology
Extending the logic of our Icelandic thought experiment – where we asked leading AI agents to build packing lists and recommend products – we tested these tools as product specialists at the bottom of the funnel, where shoppers weigh alternatives before tapping “buy now.”
We assessed four leading platforms – Perplexity’s Shop with PRO, Amazon’s Rufus, ChatGPT and Google Gemini – across three product categories relevant to a trekking adventure.
Our starter prompts were as follows:
- Backpack: I want to purchase the Fjallraven Abisko Hike 35 Travel Pack for hut hiking Iceland’s Laugavegur Trek. I won’t carry sleeping or cooking gear. Is the slightly higher weight compared to others worth the durability?
- GPS: For hiking Iceland’s Laugavegur Trek, I am looking at the Garmin eTrex 22x handheld GPS device (I want to spend less than $200) but I’ve heard adding maps can be tricky and battery life may not be optimal.
- Sunscreen: Is Neutrogena Ultra Sheer Mineral Face Liquid Sunscreen Broad Spectrum SPF 70 a good choice for a multiday hike in Iceland for someone with sensitive skin? This product offers good sun and wind protection but is it sufficiently waterproof?
We conducted in-depth research before starting with our agents, ensuring familiarity with each product’s strengths and weaknesses we could compare to the agents, and consistently used our starter prompts to project realistic objections-handling scenarios with each agent.
In each case, we wanted to evaluate:
- Technical product knowledge
- Transparency when information is limited
- Alternative product suggestions
- Objection resolution capabilities
We created a simple scoring methodology that allowed us to rate each agent for each product on a scale of 1-5 (worst to best) in each category, running multiple tests of the same prompt in each agent to overcome the inherent variability of LLM responses.
Our progressive questioning worked as follows: We began with initial product query (prompt), then follow-up technical questions and lastly objection resolution as needed. We then scored each round with whole numbers, rounding up for clarity.
The results are depicted in radars by product category:
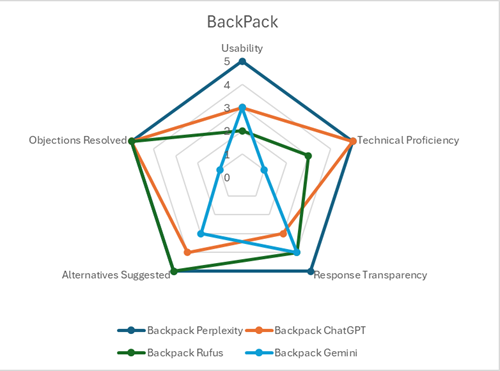
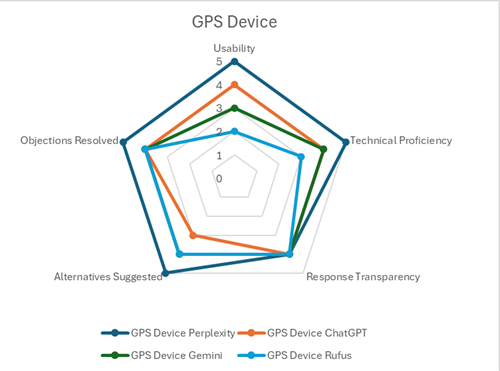
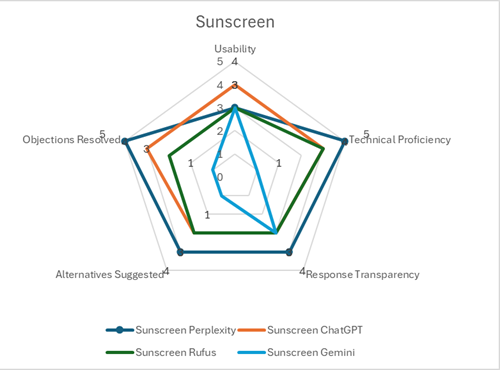
For those interested, our scoring matrix and individual scores can be seen here.
Discussion: How Agents Performed
Perplexity: The Agentic Ecommerce Leader
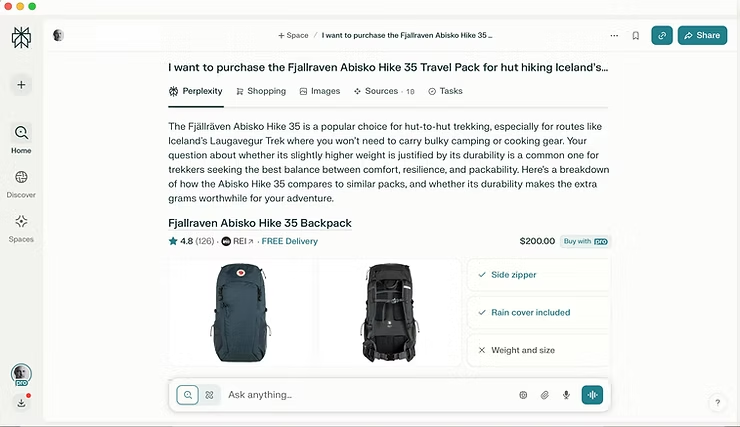
Perplexity dominated our testing with consistently impressive scores across all products (25/25 for backpack, 24/25 for GPS, and 21/25 for sunscreen).
The model’s ecommerce excellence stems from a combination of thoughtfully structured answers, contextual awareness and technical precision that made responses feel genuine and empathic. For example, it reassured us that the Fjallraven pack was purpose-designed for hut hiking, with durability outweighing its slightly heavier weight.
Perplexity stands out with user-friendly content:
- For each product query, responses were structured with tabs separating the answer, shopping options, sources and even notes about answer creation.
- When comparing alternatives, Perplexity excelled at building detailed tables that made differences apparent, as with the backpack, by featuring the Osprey Talon 33, another top choice we already had in mind.
- Ultimately, what truly distinguished Perplexity was its contextual intelligence that anticipated real-world usage scenarios.
Going the extra mile: when handling the GPS objections, Perplexity didn’t just suggest extra batteries — it specified three sets of lithium batteries (starting with a fresh pair), demonstrating a profound understanding of our scenario.
For technical challenges like map management, Perplexity provided help-desk quality guidance, even differentiating between PC and Mac workflows for sim card transfers — a thoughtful detail that would prevent user frustration in the field.
Perplexity also excelled at balancing honesty with helpfulness. For the sunscreen, it acknowledged that our chosen product wasn’t water-resistant and could be problematic during strenuous hiking in unpredictable Icelandic weather.
Instead of stopping there, it curated a detailed comparison of five mineral-based alternatives specifically chosen to accommodate our sensitive skin requirement.
Perhaps most impressive were Perplexity’s consistently spot-on “related questions” sections, which typically anticipated many natural follow-up questions and created a conversational flow that felt intuitive rather than mechanical.
The only notable weakness appeared during the sunscreen test, where we experienced inconsistent visual presentations across multiple searches, creating minor dissonance in an otherwise stellar experience.
ChatGPT: A Strong Contender
ChatGPT delivered consistently solid performances across our tests (20/25 for backpack, 19/25 for GPS, 18/25 for sunscreen), demonstrating strengths in organization balance, and clear communication, though lacking Perplexity’s polish.
From our initial backpack question, ChatGPT immediately grasped our context, validating our weight concern and similarly recognized the benefits of durability for a few extra ounces. Its responses consistently featured a clean structure with helpful headers and bullet points that made information easy to digest.
For the GPS device, ChatGPT provided chunky paragraphs that directly addressed our map management and battery life questions without overwhelming detail, while for the sunscreen, it efficiently identified that our chosen product’s lack of water resistance would be problematic for our multi-day hiking scenario.
ChatGPT particularly excelled at balanced assessment, providing nuanced discussions of product tradeoffs rather than one-sided recommendations. For the backpack, it also offered a robust comparison with the Osprey model, though without the tabular format that would have made comparisons even easier to process.
The experience wasn’t without flaws. ChatGPT’s text formatting sometimes felt bulkier and less streamlined than Perplexity, and its presentation of alternative products was less robust than other platforms, requiring more scrolling to find prices.
We also found its use of emojis distracting and inappropriate for a shopping context, degrading an otherwise professional experience.
We noted with interest ChatGPT’s transparency about its product selection process, with a subtle link explaining that “ChatGPT chooses products independently.”
This disclosure suggests OpenAI is carefully navigating the ethical considerations of product recommendations as it evolves its ecommerce capabilities.
Amazon’s Rufus: Competent but Constrained
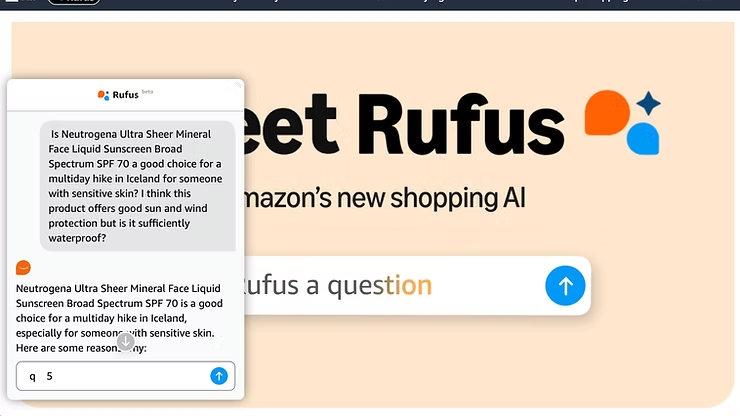
Rufus maintained consistent but unremarkable scores across our tests (19/25 for backpack, 19/25 for GPS, 16/25 for sunscreen), demonstrating solid product knowledge while being held back by interface limitations and inconsistent recommendation quality.
Where Rufus truly shined was in its integration with Amazon’s vast product ecosystem. For example, with the GPS device, Rufus proactively displayed relevant accessories like extra batteries, cases and screen protectors that complemented the main product.
Rufus provided accurate and balanced information:
- For the backpack, it highlighted the Fjällräven’s durability and organization benefits while acknowledging our weight concern.
- With the GPS device, Rufus recognized both the popularity of the eTrex 22x and suggested practical workarounds like pre-loaded SD cards.
However, compared to the other LLMS, the chatbot’s miniaturized format severely limited its utility. The small interface window constrained answer depth and breadth, making it difficult to present comprehensive information in an easily digestible format.
Navigation between Rufus and product pages felt disjointed, creating a fragmented experience that interrupted the decision-making flow.
A deeper limitation with Rufus is a lack of conversation history, preventing buyers from returning to continue their research over multiple sessions, a common pattern for significant purchases.
This limitation, combined with inconsistent recommendation quality (particularly evident in the sunscreen test where alternatives were presented without clear organization or rationale), undermined Rufus’s otherwise solid performance.
Amazon’s goal is always about increasing average order value via product recommendation. In this specific context, that strategy may be counterproductive by decreasing the quality of information related to the shopper’s main interest.
Google Gemini: Promising but Problematic
Gemini struggled across our tests, hampered by an overly cautious approach, interface issues and critical factual errors that undermined its credibility as a shopping assistant.
The most glaring issue was Gemini’s hesitant tone, which failed to project the confidence necessary in a sales context. For the backpack, Gemini repeatedly punted to “personal preference” rather than providing clear recommendations based on our scenario.
When discussing the GPS device, Gemini described it as “appearing to be a reasonable option” or something that “could work” for our intended use — hedging language that creates doubt rather than confidence at critical decision points.
Gemini’s interface presented significant friction. The system offers two distinct information modes: basic answers that often lack sufficient detail, and “deep research” reports that swing to the opposite extreme with overwhelming information density.
This “Goldilocks problem” meant Gemini struggled to find the right balance of detail for effective shopping guidance. While deep research impresses with its ability to analyze dozens of sources, few shoppers are likely to accept a delay of 3-5 minutes for the results.
Most concerning was Gemini’s factual error with the sunscreen test, where it incorrectly identified our chosen product as water-resistant when it wasn’t — confusing it with a similarly named but compositionally distinct Neutrogena product.
This mistake highlights the dangers of AI confidence without accuracy, especially when product names differ by just a few words (suggesting also the increasing importance of catalog optimization for etailers).
Despite these issues, Gemini did offer insights the other assistants missed. For the GPS device, it uniquely observed that hikers often also use mobile apps for navigation and suggested bringing a spare power pack — a practical consideration relevant to our scenario – as these services rapidly drain barreries.
This type of contextual thinking shows the potential for deep customer empathy, though it wasn’t consistently applied across all products.
Takeaways on Agentic Shopping
Today’s AI shopping assistants show impressive capability as on-demand product specialists, particularly for answering technical questions and comparing alternatives.
Perplexity leads the pack with its superior organization and contextual intelligence, while Rufus offers the most seamless integration with an actual purchase process. ChatGPT is just entering the arena, while Google feels like it is lost on the way to the competition.
That said, all the assistants we tested show limitations. Interface constraints, inconsistent detail depth, and occasional factual errors (such as with similar product names) highlight that these tools remain supplements to, rather than replacements for, human expertise.
- For shoppers: AI shopping assistants offer significant value in accelerating research and narrowing options. This suggests using these tools to gather technical information and identify alternatives, while verifying critical details before purchase.
- For retailers: The future means competing not just on price but on expertise. Merchants who succeed will embrace AI discovery tools, strengthen loyalty loops (and excel at fulfillment) – so that AI agents recognize and recommend their goods to buyers.
The best news? This technology is advancing rapidly, meaning the gap between AI and human product specialists will only continue to shrink.
Learn How to Master Agentic Ecommerce
Agentic shopping is rapidly transforming ecommerce landscape. A recent white paper from NewEcom provides a strategic roadmap for AI integration and reveals the executive competencies required to successfully navigate this revolution.